
Learn how a data leader at a large enterprise created a purpose-built platform for data teams to address bad data through end-to-end data observability and cross-platform pipeline traceability for ultimate data reliability.
Creating an Enterprise Approach to Data Quality and Data Reliability
Enterprises are defined by complexity. GE Aerospace, where I led enterprise data & analytics and spent much of my career, is a prime example. GE Aerospace has several products and services – from jet engines to flight operation software, thousands of employees across the globe, and complicated supply chains. Just one engine has an inventory of tens of thousands of parts and numerous suppliers.
Like every enterprise, this complexity extends to the data that underpins it. At GE Aerospace, we managed petabytes of data, dozens of platforms, and thousands of data pipelines. And like every enterprise, we went to great lengths to turn this data into a driver for the business. We invested in a modern data stack, data governance, self-service analytics, and programs to help foster a data-driven culture. And like every enterprise, our efforts were continually undermined by data reliability and quality issues.
Bad data is the Achilles’ heel of enterprise data teams. It erodes trust, leads to poor decision-making, and hurts efforts to become data-driven. Although bad data isn't a new problem, it has never been solved in a way that works for the modern enterprise. Data teams either rely on manual effort or employ solutions that only address part of the problem.
To effectively address bad data in the enterprise, you need to first understand enterprise environments, how they work, and how complexity defines them. Drawing from my experience testing and refining a new approach to improving data quality and data reliability at GE Aerospace, I founded Pantomath. With Pantomath, we have created a platform purpose-built for enterprise data teams. The platform is architected on the pillars that enterprises must have to ensure trusted data in complex environments: end-to-end data observability and cross-platform pipeline traceability.
A complex problem
In complex enterprise environments, the symptoms of bad data are often miles apart from the root cause – and the impact is far-reaching and difficult to track. A missing data issue within a Tableau dashboard is rarely a dashboard problem. Most of the time, something went wrong as the data moved through the pipeline. Finding the root cause, mapping the impact, and painstakingly reverse-engineering the issue when there are hundreds of potential failure points can take days or even weeks. All the while, data consumers are losing trust in the data, making decisions on bad data, or turning their backs on data altogether.
Untangling data issues manually is a time-consuming and inefficient process. Manual approaches strain data teams and become untenable as data volumes grow and use cases expand. Often larger enterprises resort to employing large offshore teams just to address data issues.

In recent years, data observability solutions have emerged to bring automation to the detection of data issues. The problem is that these tools only detect the symptoms of bad data. They look at that data at rest, once it has landed in the warehouse – largely ignoring the data as it moves through the data pipeline across different data platforms and tools where the majority of issues occur. The root cause of data issues is also most often in the data pipeline. Without visibility into the interdependencies and relationships across the data pipeline, the heavy lift is still on data teams to troubleshoot and remediate issues. Alerts function like a check engine light rather than a diagnostic.
I saw the limitations of these approaches as a data leader at a large enterprise. I understood that neither was effective enough to effectively solve the “bad data” problem. I also recognized that a blueprint for the solution exists. Companies like Datadog and Splunk have taken automated, end-to-end approaches to observability and applied them to software development and machine data. I realized that enterprise data teams needed a similar approach to data quality and data reliability.
Complex problems require end-to-end solutions
To solve the bad data problem in the enterprise, we started by addressing complexity. In an environment where there are hundreds of potential failure points, the only viable solution is to look at the entire data pipeline – from production to consumption.
To that end, we architected Pantomath for end-to-end data observability. The platform automatically monitors data at rest and in motion as it moves through every platform and system across the data pipeline. With end-to-end data observability, Pantomath can detect issues no matter where they occur. Detection doesn't end at the dashboard, it extends to the failed transformation job where the issue started.
Automatically detecting issues with end-to-end data observability is the critical first step. The next step is to trace the impact of those issues and enable data teams to fix the problems with minimal disruption to data consumers and minimal data downtime.

To address this, we architected Pantomath with cross-platform pipeline lineage. Cross-platform pipeline lineage goes beyond data lineage to trace the flow of data across every stage of the data pipeline. A delayed or failed pipeline job or one that doesn’t start in time creates a domino effect that impacts downstream jobs and analytics. With cross-platform pipeline lineage, data teams have a map of everything that went wrong, the impact, and a clear understanding of how to set everything right. No need to painstakingly reverse-engineer data issues to identify the root cause and understand the impact.
In a complex enterprise environment, where a bad report or dashboard is only the tip of the iceberg, data teams need complete visibility. Only automated, full end-to-end data observability and cross-platform pipeline traceability can ensure healthy and reliable data, remove the weight of bad data, and improve trust.
Built for enterprise teams
As powerful as end-to-end observability and cross-platform pipeline traceability are, we understood that an enterprise platform has to work for enterprise teams. Enterprise data teams are already working with numerous tools and priorities. With that in mind, we designed Pantomath to tell data teams exactly what they need to know to remediate data issues with as little friction as possible.
For example, an alert is only useful if it is actionable. Meaningless or low-priority alerts quickly lead to alert fatigue. To that end, Pantomath only generates an alert when an issue is actionable and impacts analytics downstream. Each alert includes all of the information that a data engineer would need to quickly access and remediate an issue, including the root cause, the resulting impact, and the best process for remediation. Actions are recorded in the same alert, helping data teams to work asynchronously to fix complex problems.
At every step, we designed Pantomath with enterprise data teams in mind. From being able to visualize complex data pipelines to enabling cross-functional collaboration and reporting on the health of the organization's data, our enterprise experience extends to every part of the platform.
Introducing Pantomath
I’ve been in your seat. I’ve seen the negative impact that bad data has on enterprises, undermining all of your efforts to be data-driven. I’ve also seen the shortcomings of current solutions. Manually addressing bad data issues is untenable in enterprises where it can take days or weeks to remediate an issue, and data observability solutions simply don’t go far enough.
With Pantomath, we’ve architected a platform from the ground up to give data teams the automation and end-to-end visibility they need to quickly identify and remediate data issues. I’m excited to bring Pantomath to market and help data teams like those at Lendly, Coterie Insurance, G&J Pepsi Bottlers, and Paycor overcome the hurdle of bad data and reach their true data-driven potential.
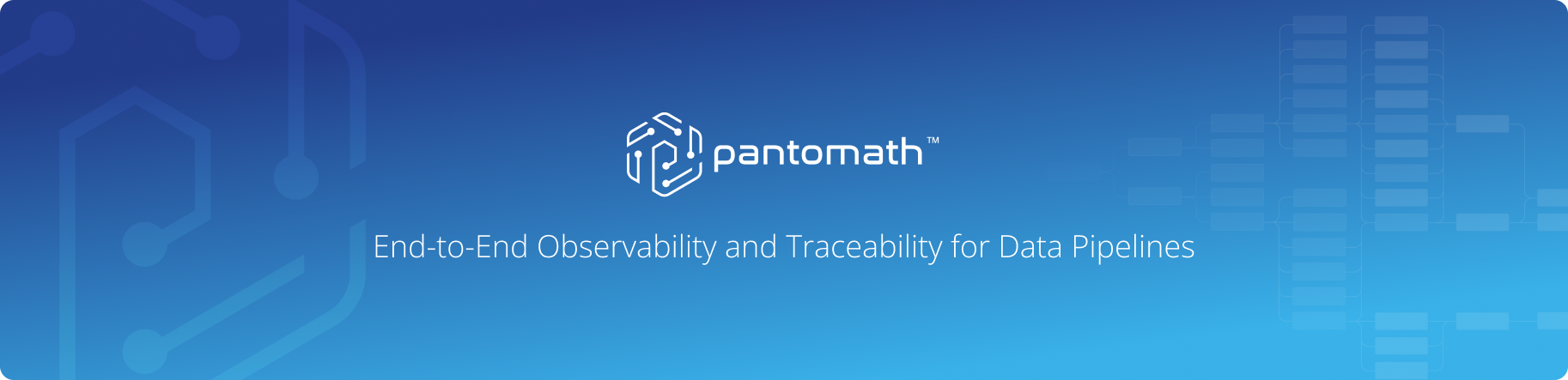
Keep Reading
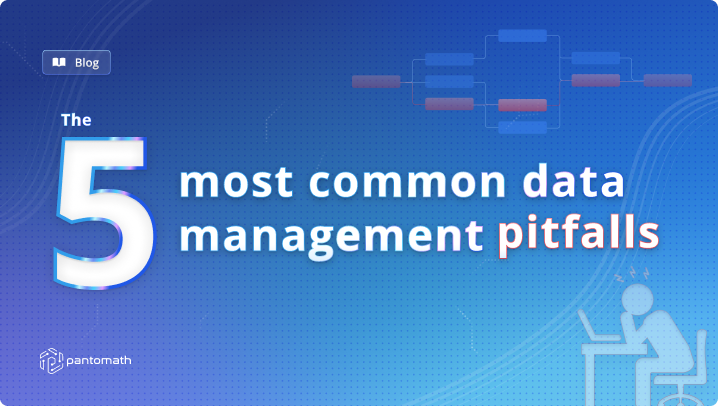
September 18, 2024
The 5 Most Common Data Management PitfallsThere are five common mistakes that teams often make on their journey toward data observability. Learn how to avoid them!
Read More
August 7, 2024
10 Essential Metrics for Effective Data ObservabilityYou can’t simply implement data observability and then hope for the best. Learn about the top 10 essential metrics to make your business thrive.
Read More
July 26, 2024
The Evolution of Data Quality: Why Data Observability is KeyData quality has a long history, dating back at least to mainframes in the 1960s. Learn about the path Data Quality has taken, and where it's headed.
Read More