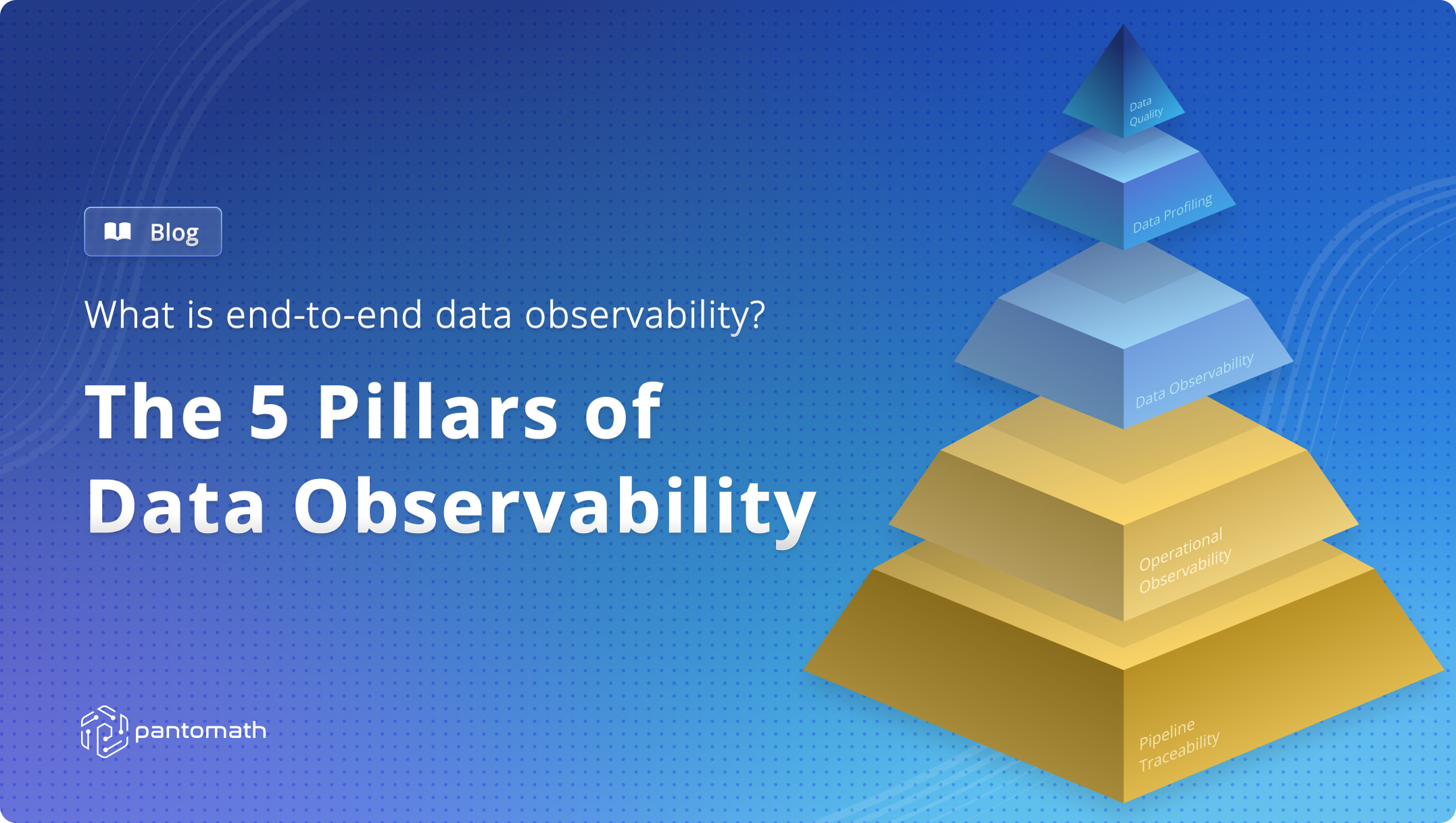
Take a deep dive into the The 5 Pillars of Data Observability, and End-to-end data observability.
The 5 Pillars of Data Observability
Poor data quality costs enterprises millions each year in data downtime, poor decision-making, and time spent resolving issues. Distributed data landscapes, diverse datasets, and complex data pipelines make addressing data quality and improving reliability more challenging than ever.
Traditional data quality and monitoring tools have existed for decades but are insufficient in these dynamic environments where potential issues aren’t yet understood and detected. Data observability emerged as a modern alternative to these tools. The goal of data observability is to provide up-to-the-moment status updates on the health of an organization’s data.
While an improvement over traditional tooling, data observability only looks at data at rest, once it has landed in the warehouse, lake, or lakehouse – ignoring the rest of the data pipeline. This limited view misses key issues, like data latency, data movement, and other problems that occur when data is in motion. By only looking at data at rest, data observability also doesn’t identify the root cause of data quality and reliability issues.
In complex data environments, data teams need full end-to-end observability and traceability. Only with a complete understanding of the entire data pipeline can enterprises be confident in the quality of their data and the reliability of their data pipelines.

Introducing end-to-end data observability
The data pipeline is the backbone of the entire data environment. It starts at the production layer (ERPs, application databases, etc.) and runs all the way to the consumer layer (the BI platforms, visualization tools, etc). Along the way, that pipeline interacts with numerous tools and frameworks, including data integration, data orchestration, data warehousing, ML ops, and more. Things can – and will – go wrong at any point in this journey, making pinpointing issues and their causes extremely challenging.
Making things more complex, the teams supporting data operations are living in silos and bound to view the pipeline from each technology's lens of the world. Data teams aren’t able to see how different pieces of the puzzle fit together. This makes detecting and resolving issues a painstaking process. Multiple teams must work together to manually reverse-engineer complex data pipelines across different platforms in the hope of determining the root cause and impact of the issue.
End-to-end data observability builds on data observability to include deeper visibility into the data pipeline and data in motion. End-to-end data observability monitors the data and jobs that run at every point in the data pipeline from the production layer to the consumer layer and at every touchpoint in between.
In this blog, we will define the five pillars of end-to-end data observability. We’ll start with the three pillars that are generally covered by existing data quality and data observability tools:

Data Quality: Monitoring data quality and data values
Data Profiling: Monitoring data distribution and segmentation
Data Observability: Monitoring data at rest for missing or stale data
Then we will dive into the two pillars that build on data observability and provide the greatest value for improving data quality and reliability:

Operational observability: Monitoring data in motion for pipeline issues like data latency, data movement, jobs that haven’t started, and job failures
Pipeline traceability: Cross-platform data pipeline linage, including jobs and datasets
Observing data at rest
The first three pillars observe data at rest. These pillars ensure that the data looks as expected when it has landed in the warehouse, lake, or lakehouse.
Data Quality
Data quality anticipates specific, known problems in the data. This includes data validation checks, reconciliation checks, accuracy checks, and other business rules. These checks and rules ensure that the data is correct and that the values are accurate.
Data Profiling
Data profiling looks at the distribution and segmentation of the data. This helps to detect issues at the field level and includes monitoring the statistical measurements of counts, mean, median, mode, min/max values, standard deviations, and quantities of each column in a dataset.
Data Observability
Data observability monitors a dataset's volume and freshness. Data observability ensures that the data is complete and that the data was refreshed at the right time through anomaly detection.

Operational Observability
Operational observability monitors the flow of data in real-time, while it is in motion. It also monitors the jobs that must run to ensure that the data flows correctly through the data pipeline. This pillar detects issues that can’t be detected by observing the data at rest, including:
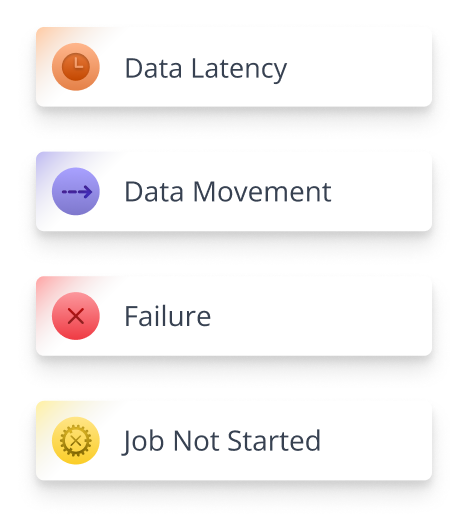
● Data latency: Detecting whether the data is on time as it moves from one transformation touchpoint to another as it is being transformed
● Data movement: Detecting whether the data is complete and all the records have made it from one transformation touchpoint to another as expected
● Job failure: Detecting job failures and their downstream impact in real-time
● Job not started: Detecting when a job doesn't start at its scheduled time to prevent downstream jobs from running without correct or complete data
In addition to detecting issues as they occur in the pipeline, operational observability troubleshoots incidents with real-time event logs, error messages, historical trends, and automated root cause analysis. By doing so, operational observability correlates the information needed to provide data teams with recommendations on how to resolve issues most effectively.
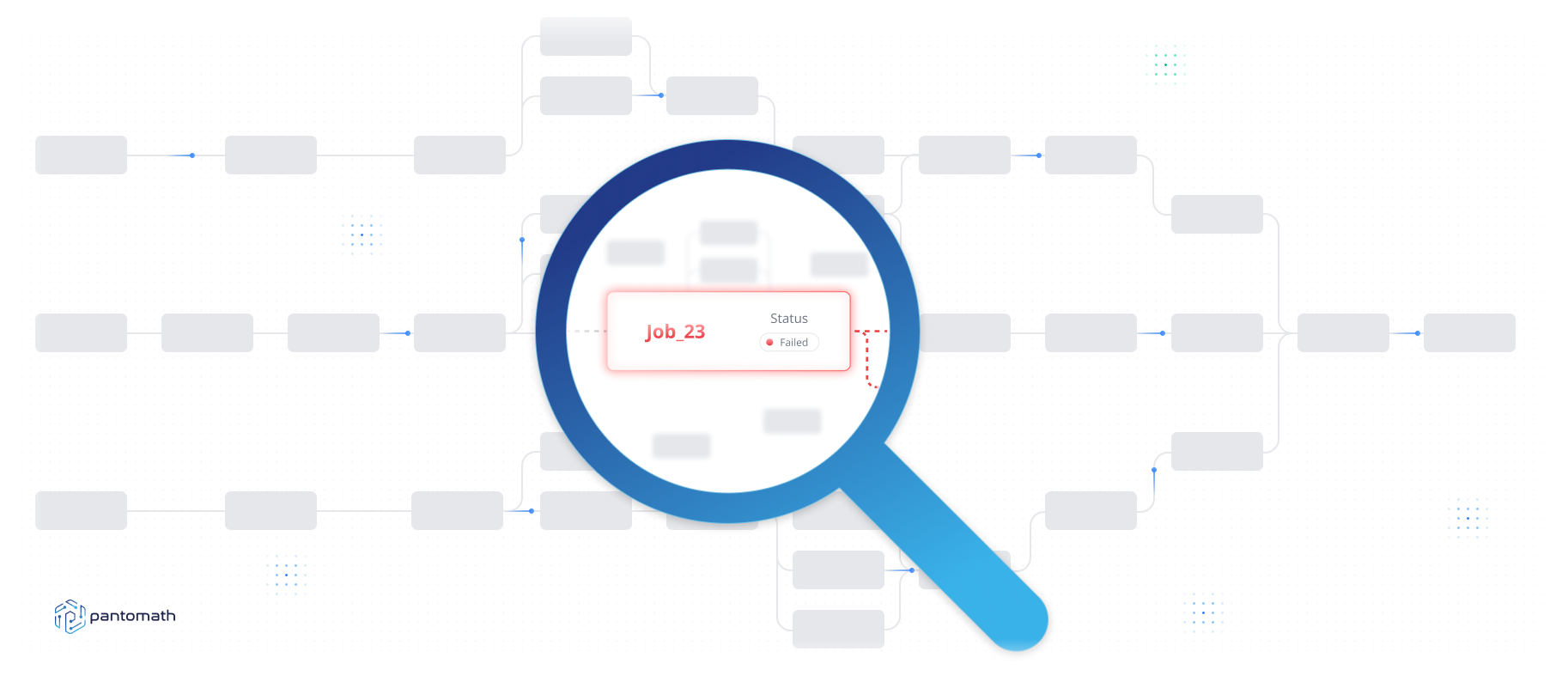
Pipeline Traceability
By only looking at the datasets in the data warehouse, data teams aren’t given the visibility they need to effectively diagnose issues. Data lineage alone can’t provide a full understanding of what is occurring across the data pipeline. As a result, data teams must painstakingly reverse-engineer complex data pipelines to attempt to resolve issues.
Pipeline traceability pinpoints the root cause of issues that occur at any point as the data flows across the data pipeline. This enables data teams to resolve problems faster and without the time-consuming manual effort that can take as much as 40% of a data engineer's time.
Pipeline traceability – or end-to-end cross-platform "pipeline" lineage – provides deep application-level technical job lineage combined with data lineage to give a complete view of the data pipeline. Every interdependency and relationship is fully mapped and kept up to date with auto-discovery of every data pipeline across the data ecosystem. The end result is faster resolution, less data downtime, and greater reliability.
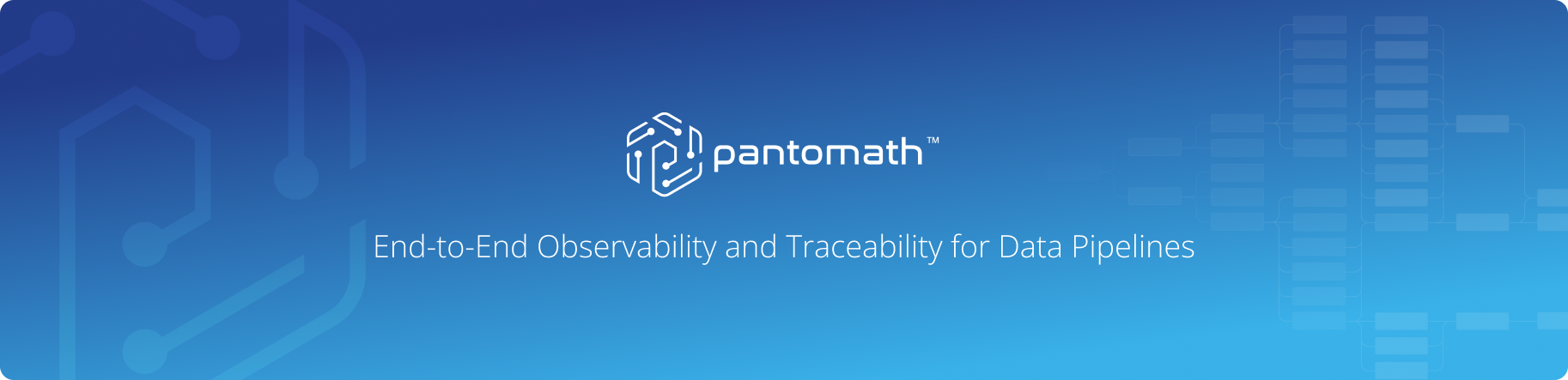
Learn more about end-to-end data observability
End-to-end data observability provides visibility into the entire data pipeline and pinpoints the root cause of data issues. With end-to-end data observability, data teams have the visibility they need to meaningfully improve data quality and ensure reliability in distributed data landscapes with diverse datasets and complex data pipelines.
If you are concerned about data quality and reliability and would like to learn more about end-to-end data observability, schedule a demo with our expert team. We’re excited to share details about our modern and innovative cloud-based solution.
Keep Reading

February 27, 2025
Data in Motion Series, Part 2: Real-Time Pipeline Monitoring Best Practices and ROIUnderstanding data in motion is crucial. But why, exactly? Ultimately it comes down to your bottom line –– you can prevent business disruption as data flows through your systems.
Read More
January 8, 2025
Data in Motion Series, Part 1: What is Data in Motion?Traditional platforms treat data as a static resource which no longer works for today's data demands. Learn more about the importance of monitoring BOTH data at rest and data in motion.
Read More
November 4, 2024
Why Data Observability is Essential for Generative AI (GenAI)Discover the real-world benefits and how companies can optimize GenAI applications by implementing robust data observability practices.
Read More