
Learn about the data lineage challenges and how data pipeline traceability can provide a complete understanding of the data's journey to improve quality and reliability.
Overcoming Data Lineage Challenges with True End-to-End Data Observability
Data pipeline lineage and why it is a necessity for data quality and reliability
To trust in the quality and reliability of your data, you need to understand its complete journey. Only with a complete understanding of the data's journey can you pinpoint the root cause of issues and mitigate their impact.
This is a well-accepted concept, which is why data lineage often goes hand in hand with data observability. The thinking goes that data lineage maps the data’s journey and provides the information needed to improve data quality and reliability. In reality, however, data lineage is not enough.
On its own, data lineage only tells you about a slice of the journey. Data lineage can tell you where the data came from, how it changed, and where it is used. For example, Table 1 is joined with Table 2 to create Table 3. However, before the data is consumed, it passes through numerous tools and frameworks. Understanding the data pipeline, all platforms, and systems where the data is integrated, processed, and transformed along the way is often more critical to data quality and reliability. Because these systems are most often the reason that things go wrong and where the most serious impact occurs.

Data lineage can’t help you pinpoint issues that happen as the data moves through the pipeline or the impact on the pipeline itself. Data lineage can't tell which data ingestion, transformation, orchestration, or BI job moves or transforms the data. Data lineage lacks technical and operational depth and is missing the granular job details of how the data goes from one table to another. To truly understand the data's complete journey, you need pipeline lineage. You can meaningfully improve data quality and reliability only with data and pipeline lineage.
The challenges and limitations of data lineage
As the name suggests, data lineage looks at the data itself: where it came from, how it has changed, and where it is being surfaced to end consumers. Things can go wrong with the data, but the more serious and difficult-to-solve issues occur within the platforms and systems that make up the data pipeline.
A single problem in the data pipeline—a delayed job, a failed job, or latency—can compromise the integrity of your entire data flow. More often than not, these pipeline challenges are the root cause of data issues in the consumer layer.

To effectively remediate these pipeline challenges, data teams need to know the root cause and how the rest of the pipeline was affected. A failed or delayed job can create a domino effect, wreaking havoc on the rest of the pipeline. Data lineage can’t provide visibility into the interdependencies and relationships across the data pipeline. As a result, even with data lineage, the heavy lifting is still on data teams, who must reverse engineer the pipeline to find the root cause and diagnose the impact.
Adding more complexity to the problem, data engineers rarely work with all the platforms and systems in the pipeline, meaning remediation requires collaboration across teams. With dozens or even hundreds of potential failure points within a given data pipeline, data teams need greater visibility into the data pipeline than data lineage provides.

Pipeline traceability
Cross-platform pipeline lineage is the only way to truly understand the data’s journey. Only through this can you see what went wrong at every stage of the pipeline, from the source systems to the reports and at every hop in between. At Pantomath, we call this combination of data lineage and deep, application-level job lineage end-to-end pipeline lineage or pipeline traceability.
With Pipeline Traceability, you can do two things that are critical for data quality and reliability:
● Quickly and accurately identify the root cause of issues: Data teams can pinpoint the root cause. Whether the root cause was in the pipeline, like a failed job, or happened in the consumer layer, like an improper schema change, the data team knows exactly what went wrong and how to fix it. This enables data teams to resolve problems faster and without the time-consuming manual effort that can take as much as 40% of a data engineer's time.
● Understand the complete impact of those issues and enable resolution: Pipeline Traceability maps every interdependency and relationship of every data pipeline across the ecosystem. With Pipeline Traceability, data teams can fully understand the impact of issues—not only what reports and dashboards have been affected but also which jobs need to be re-run to resolve the issue and bring the pipeline back up and running with refreshed data.
Pipeline traceability and end-to-end data observability
Pipeline Traceability is the foundation necessary to achieve comprehensive end-to-end data observability. With end-to-end data observability, data teams know when things go wrong, what went wrong, and what was impacted. At a time when data is becoming more distributed and data pipelines are becoming more complex, end-to-end data observability is critical for improving data quality and ensuring reliability.
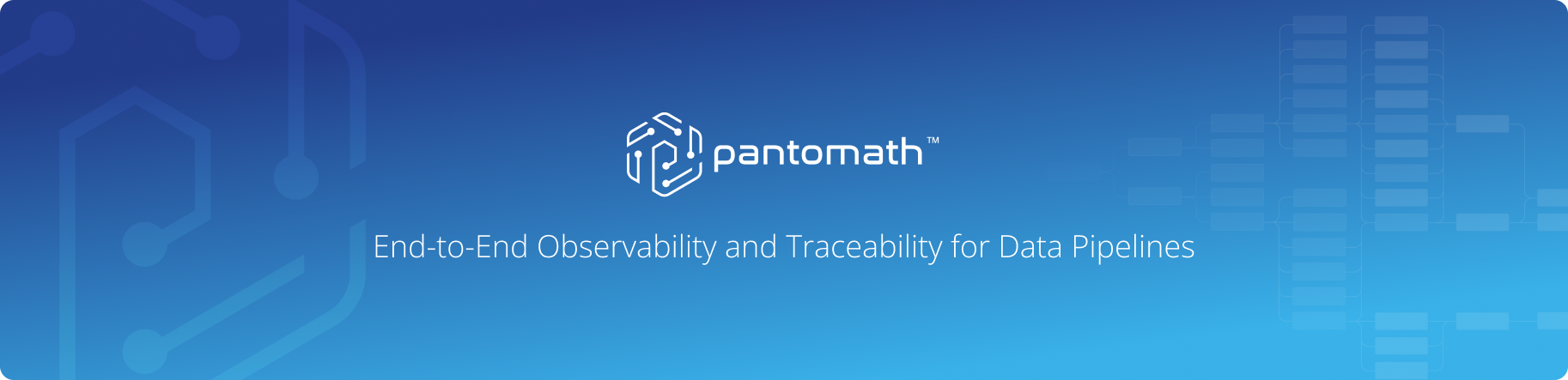
If you're interested in improving data quality and reliability and want to explore end-to-end data observability more, arrange a demo with our expert team. We can’t wait to show you our innovative cloud-based solution.
Keep Reading

June 2, 2025
Pantomath and Alation Expand Partnership to Deliver Comprehensive Data TrustThis collaboration builds on the newly launched Alation Data Quality (DQ) Agent, further strengthening the ability of enterprises to govern, observe, and trust their data across the entire ecosystem.
Read More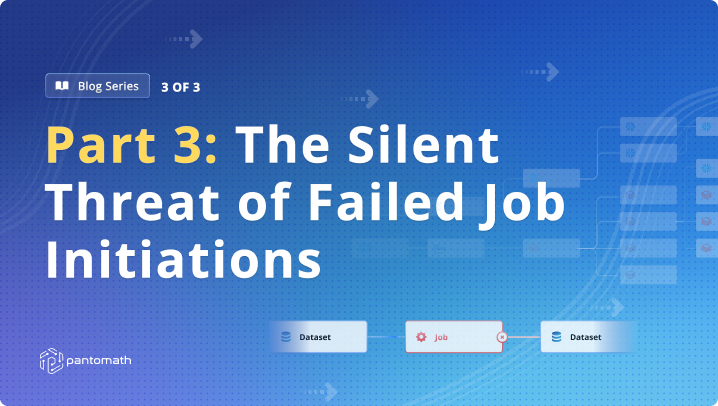
May 19, 2025
Data in Motion Series, Part 3: The Silent Threat of Failed Job InitiationsWhile much attention focuses on data quality issues and pipeline failures, the simple failure of a job to start can trigger catastrophic downstream effects.
Read More
May 13, 2025
SLA Accuracy in ServiceNow: Are You Tracking the Signal or the Noise?Here’s the uncomfortable truth: the way most organizations manage data incidents in ServiceNow is broken. Pantomath's Somesh Saxena gives his thoughts.
Read More